TRACE
Bloomberg’s New Machine Learning Hub for Simpler Experiment Tracking, Documentation, and Discoverability
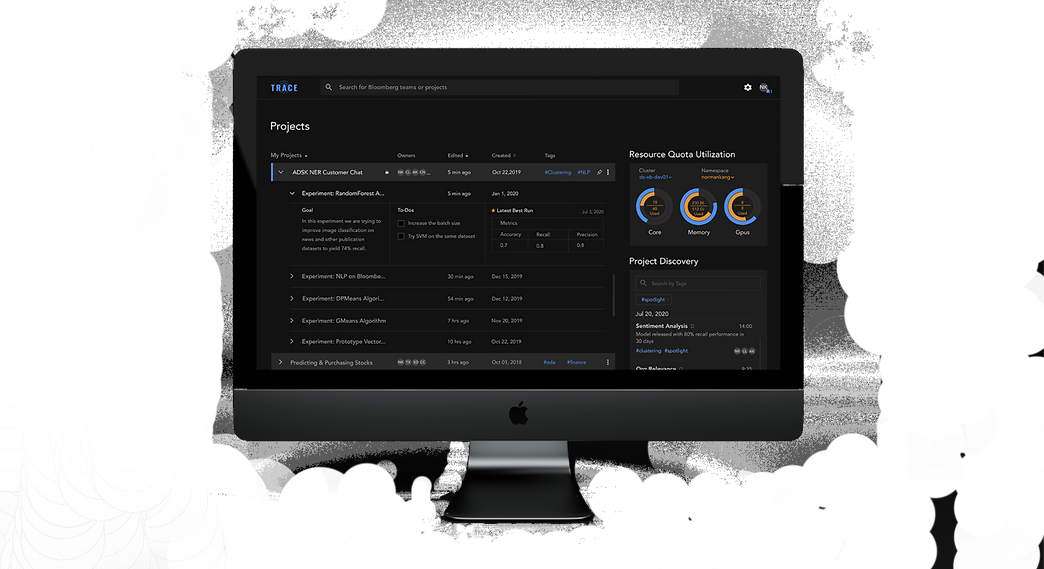
"This is so tedious"
The recent growth of machine learning magnifies an inherent pain point: scalability.
Machine learning (ML) is rapidly expanding and being applied to nearly every facet of our client Bloomberg’s business. The tedious, manual nature of ML engineers' workflow is amplified when they apply ML at scale.
​
Since Bloomberg had no existing tools available that addressed their unique challenges, we decided to design a desktop app from the ground-up that introduces automation, streamlines documentation, and improves collaboration.
In the 8 months, I was a designer who led the research portion during the first half of the semester and designed and iterated on interactions and UI during the second half of the semester.
Duration
8 months
Role
UX Designer
Responsibilities
Research, Interaction Design, UI Design
Team
Amy Lu, Neha Chopade, Danielle Shoshani, Norman Kang
Solution

Trace is an all-in-one centralized platform that is designed specifically to improve upon three integrated components: tracking, documentation, and discoverability. It stitches together flows that are currently segmented into one cohesive workflow.
Tracking is writing down all the necessary details of an iteration to test out their hypothesis
Documentation is keeping track of important iterations in a structured way for colleagues and managers
Discoverability is the ability to be up-to-date with their teammates and other teams’ current and previous work
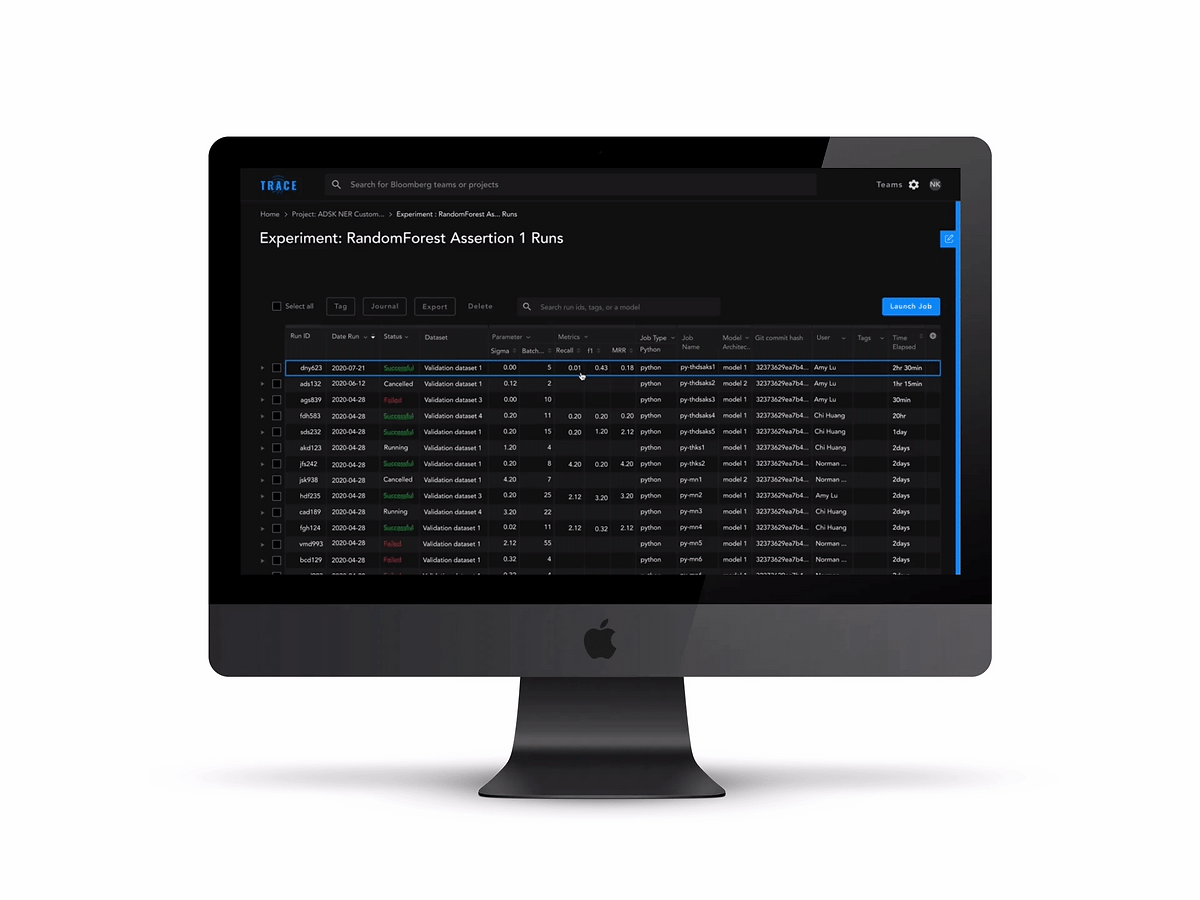
COMPREHENSIVE TRACKING
Trace allows ML engineers to track all the necessary artifacts to reproduce the different iterations of their work, along with their in-the-moment thought processes.
​
When ML engineers generate and compare different iterations, they may gain an insight that informs their next step. If the insight isn't tracked in that very moment, the thought process that gives the iteration its meaning and value is lost.
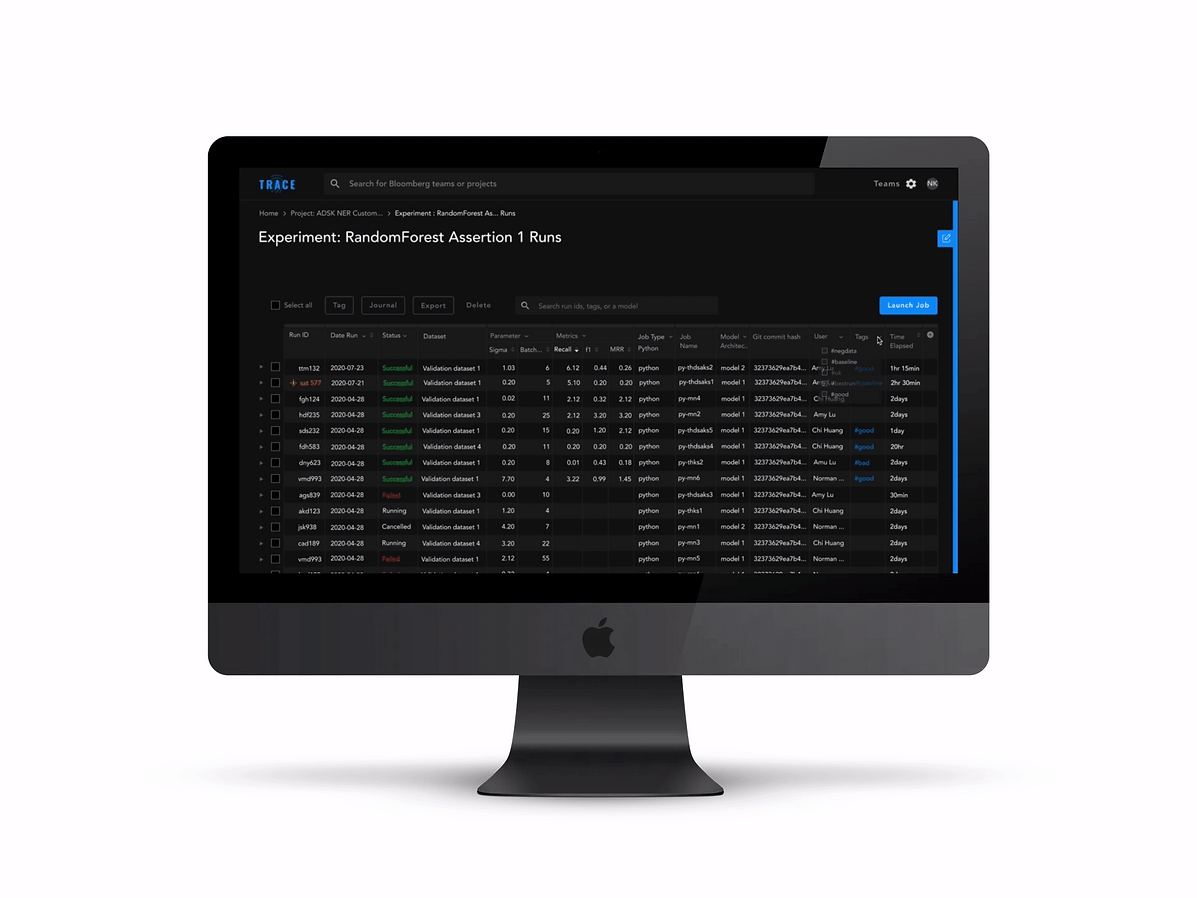
EASY TRANSFER TO DOCUMENTATION
Trace automated the documentation process by allowing the engineers to easily transfer the details of their work.
​
Oftentimes the most tedious part of documentation isn’t the actual writing: it’s having to manually build tables, transfer data, and record the details of the iterations they tested out. The tediousness of this manual task increases the probability of poor documentation and wasted time and effort.

STRUCTURED WAY FOR DISCOVERABILITY
Trace reduces back-and-forth conversations by providing various ways for the engineers to discover their teammates and other teams’ work.
​
The current way for Bloomberg's machine learning engineers to discover other people's projects is through verbal means. This oftentimes causes work to be repeated and knowledge to be siloed when these verbal communications don’t happen.
Deep dive into user pain points & design opportunities
In order to help the team understand the complex domain of ML and gain enough expertise to design for our target users—Bloomberg’s ML engineers—I led the research initiatives to understand user needs and prioritize pain points.
METHODS:
100+
Participants
11
Methods
Literature Search
YouTube Videos & ML Course
Competitive Analysis
Field Research
Semi-Structured Interviews
Directed Storytelling
Workflow Codesign
Love Letter/Breakup Letter
Affinity Diagramming
Storyboards
Usability Testing
CURRENT WORKFLOW & TOOL USAGE
Through workflow codesigns, we mapped out ML engineers’ workflows, the tools that they use, and their pain points. Interestingly, there are remarkable inconsistencies in tool usage (indicated in the diagram) in two stages of their workflow—tracking and documentation. This inconsistency is evident both across and within teams.
​
The reason for such discrepancies is that the current tools that the engineers use don’t address their needs for tracking and documentation, so that forces them to come up with their own workarounds.
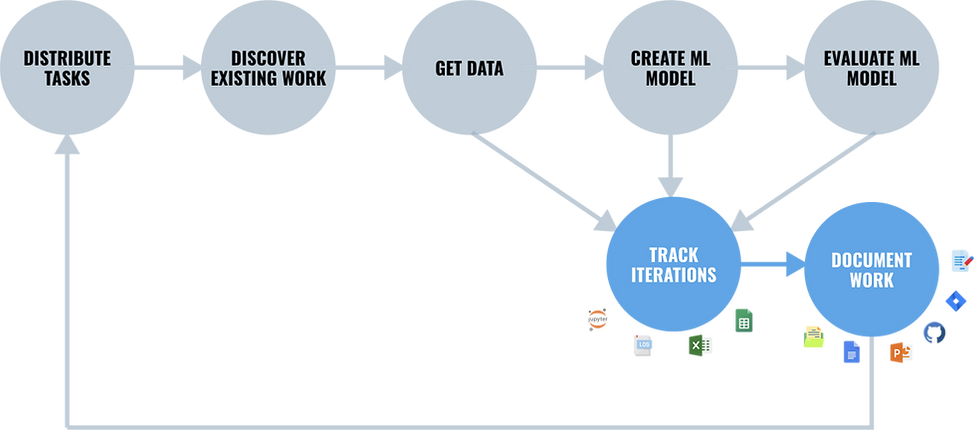
GUIDING INSIGHT:
The segmentation of ML engineers’ workflow into three independent components—tracking, documentation, and discoverability—has introduced a lot of manual work and frustration.
This is the insight that ultimately shaped the direction of our project. We’ve uncovered the interdependent relationship between tracking, documentation, and discoverability. The cascading effect of one component to the next suggests the need for an integrated platform that supports those three areas to streamline ML engineers’ experience.
​
An integrated platform founded on solid tracking could have positive, snowballing effects on the remaining aspects of their workflow, bringing them the most value.
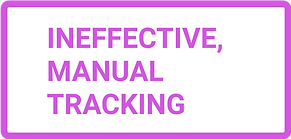
Tracking is ineffective because the iterative nature of ML and the manual aspect of tracking make it impossible to record all the important iterations.
The ML process is highly iterative and each small change can influence an iteration's performance. That coupled with the hundreds of iterations they produce on a weekly basis and needing to manually copy and paste the iterations' details, ML engineers are often forced to cherry-pick which iterations to track, leading to lost work.
Tracking is writing down all the necessary details of an iteration to test out their hypothesis

Tracking breeds inconsistencies in documentation.
Since there’s no standardized way to track their iterations, ML engineers are left to their own devices when deciding which artifacts to use for tracking. Inconsistency in tool usage in the tracking stage further breeds inconsistency in the documentation stage.
Documentation is keeping track of important iterations in a structured way for colleagues and managers
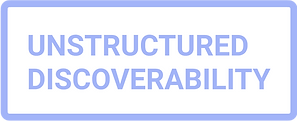
Inconsistencies in the tools used for documentation hinder the possibility for discoverability.
Because documentation is scattered in different locations, the possibility of having a more structured platform to discover others' work is prevented. The current ways Bloomberg ML engineers discover each other's work is through verbal means, such as attending sprint reviews and asking around.
Discoverability is the ability to be up-to-date with their teammates and other teams’ current and previous work
-
Lost work
-
Wasted time and effort
-
Frustration
-
Inability to understand past work
-
Unable to reproduce an iteration
-
Can't resolve disputes
-
Fail to get buy-in
-
Siloed environment
-
Duplicated work
-
Slower progress
-
Less innovative work


Ideation
(IN)VALIDATING USER NEEDS
Even though we’ve decided to design an integrated platform that supports tracking, documentation, and discoverability, we still needed to ensure that we addressed their pain points at various touch points in our design. Thus, I conducted storyboarding to (in)validate user needs to inform the details of our solution.
![]() | ![]() | ![]() |
---|---|---|
![]() | ![]() |
​
INSIGHTS:
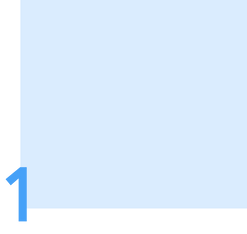
ML engineers need to quickly orient themselves and make sense of massive amount of information, as they easily generate hundreds of runs.
Quick Orientation
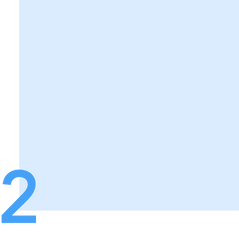
Track Thought Processes
ML engineers need to track in-the-moment the resulting insight from the specific iteration or a decision made in response to the insight.

Transfer Metadata to Documentation
ML engineers copy and paste numbers hundreds of times a week to documentation to get buy-in and make their iterations reproducible.
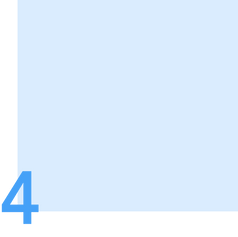
Stay up-to-date
ML engineers need a more efficient way of keeping their teammates up-to-date on their progress than engaging in back and forth conversation .
CONCEPT GENERATION FROM USER NEEDS
Now that I’ve identified important user needs that need to be addressed with our design, I mocked up various different ideas and features to address each user need. Then, I presented the mock-ups to our users for feedback.
QUICK ORIENTATION

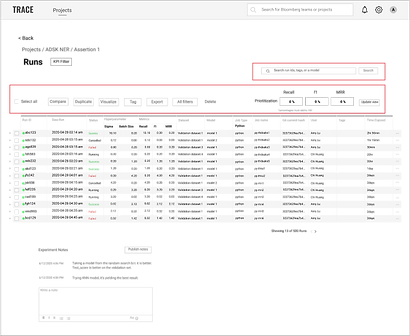
ML engineers need to quickly reorient themselves at both a high level and a detailed level.
​
Bloomberg ML engineers juggle between a maximum of five projects at once and generate hundreds of different iterations for each project on a weekly basis. Based on user feedback, at a high-level, they need to immediately know how much resources (e.g., GPU and CPU) they have left to determine whether they could launch more iterations or not. Also, at a detailed level, they need to be able to quickly sift through the different iterations.
Features included: Resource Quota Utilization and Run Status Report at the homepage. Sort, Filter, Search, and Tag on the individual project page.
TRACK THOUGHT PROCESSES
ML engineers need to track in-the-moment the insights, learnings, and next-stpes inspired by each iteration in order to accurately retrace and reproduce their steps.
​
Even though the end results/performance of each ML iteration is important, what’s even more valuable is the learnings and next steps inspired by the results of an iteration. For the purposes of documentation, reproducibility, quick orientation, and reporting and getting buy-in from stakeholders, it’s critical that ML engineers are able to retrace their steps and thought processes in a project.
Features included: A note section associated with each iteration to jot down learnings and insights; a to-do’s section associated with each project to jot down next-steps for quick orientation when working on multiple projects

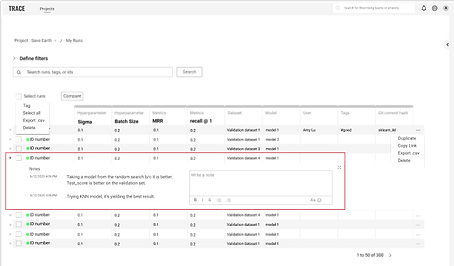
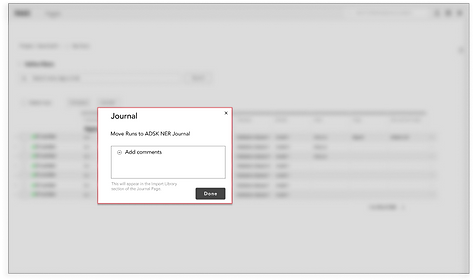
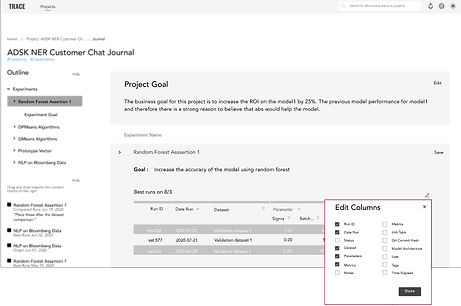
TRANSFER METADATA TO DOCUMENTATION
The process of manually creating tables and copying and pasting metadata needs to be automated.
​
We found that even though there are certain metadata that are “standards” for documentation, the metadata that ML engineers document still vary depending on their audience. Therefore, we’ve decided to automate the documentation process by transferring the “standard” metadata as default, but still provide them the autonomy to edit what data is displayed.
Features included: A button on the Iterations page that allows users to transfer an iteration's metadata to documentation. The information that’s essential to provide context (e.g., run ID, parameters, metrics, and etc.) would be displayed as default, but the user could always change what information is displayed by clicking on the “Edit” icon.

Design Iterations
After I’ve identified the specific features and functionalities to include on the screens, I presented my designs to users to gauge reactions and feedback. I rapidly iterated on the designs to ensure that the UI addresses their needs and that the flow fits their mental model.
SCREEN ITERATIONS
REDESIGN
ORIGINAL
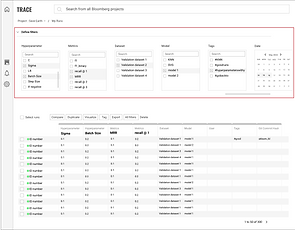

Concise display of filters frees up more real-estate for important information
​
I incorporated filters into the experiment runs table, which allows ML engineers to see changes in the runs reflected in real-time. By tightening the coupling of the input and the output, it not only dismisses the possibility of users being confused by the purpose of the filters, it also leaves a lot more real-estate to showcase the individual runs—ML engineers’ main focus.
REDESIGN
ORIGINAL


Redesigned UI elements for better usability and scan-ability.
​
Visually, I made the information hierarchy clearer (e.g., under each project resides a few experiments and underneath each experiment, there’s more detailed information pertinent to only that experiment). In addition, I moved “Resource Quota Utilization” and “Project Discovery” to the side because they’re not the main focus—they’re simply elements that the engineers would briefly check before they click on a specific project or experiment.
REDESIGN
ORIGINAL
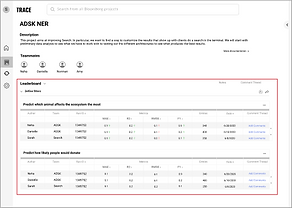
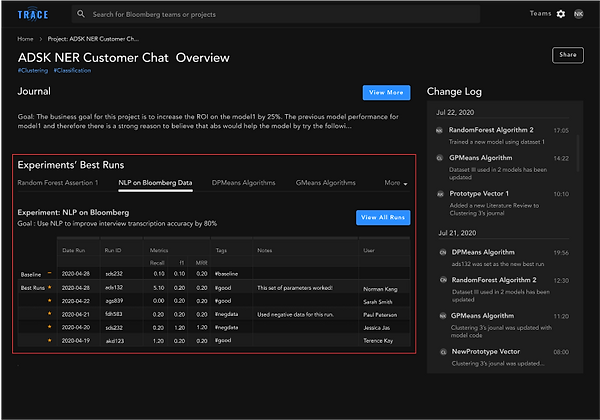
Ensured that the design not only addresses user needs, but also fits their team culture.
​
I replaced “Leaderboard,” which overly emphasized who produced the best runs in comparison to the rest of the team with “Experiments’ Best Runs,” a table that emphasized the experiments and teammates’ process without provoking competition. Bloomberg’s ML teams are collaborative, and they need to easily be in the loop of each other’s work without a sense of ranking or competition.
REDESIGN
ORIGINAL
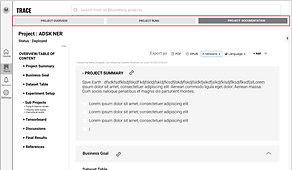
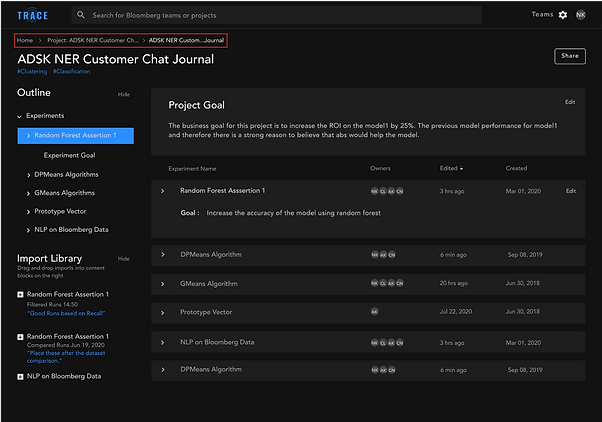
Easy-to-follow breadcrumbs that emphasize recognition over recall to allow for simple navigation
​
Breadcrumbs are extremely important to ensure that users wouldn’t get lost while using our design. I designed breadcrumbs that display sufficient information for users to quickly reenact their journeys and reorient themselves.
FLOW ITERATIONS
Based on the insights I got from user feedback and usability testing, I modified the core flow of our design. The original flow was overly complex, with a lot of overlapping functionalities between the screens, and visually identical that led to user confusion. I combined, deleted, modified, and differentiated the screens so that the flow was simpler, yet highly usable and functional.
PROJECTS
(TEAM'S PROJECTS)
ORIGINAL

HOME
PROJECTS
(MY PROJECTS)
PROJECT SUMMARY
EXPERIMENT SUMMARY
RUNS
DOCUMENTATION
HOME
EXPERIMENT SUMMARY
DOCUMENTATION
REDESIGN

RUNS
Design System
We decided as a team to design our solution in dark mode. ML engineers often use dark environments for coding, and Bloomberg ML engineers’ use a chat function daily in the Bloomberg Terminal (which is also in dark mode) for communication. We wanted to ensure visual consistency between our design solutions and the tools that they’re familiar and comfortable with.
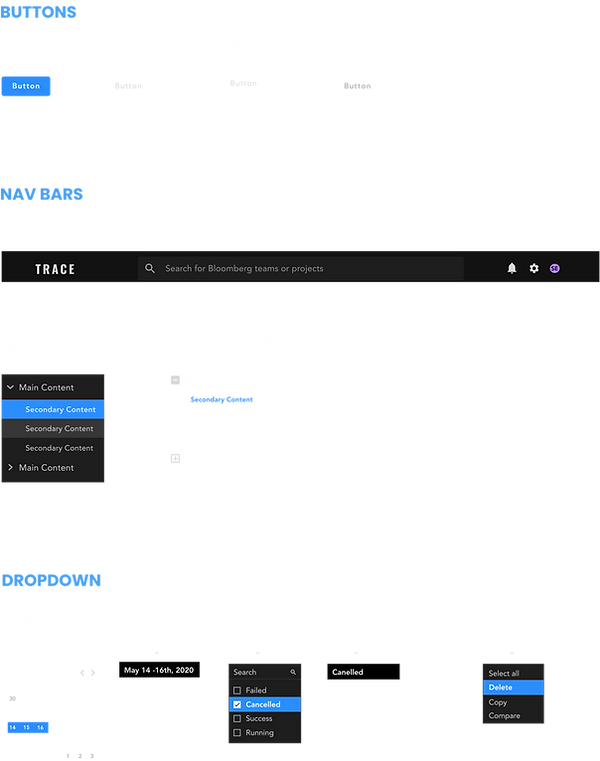
Future Visions
After we pitched our high-fidelity design to Bloomberg's senior level executives, we received buy-in for development within the next three years.
Integrate with other tools that the engineers use for further automation
​
Bloomberg's ML engineers regularly use Jira, a project management tool, and Tensorboard, a runs' performance visualization tool. If Trace could incorporate those two tools, we could further automate their workflow and streamline their experience.
Make Trace more intelligent
​
Incorporate ML to make the platform more intelligent (e.g., automate project tagging, tailor project and experiment suggestions, and etc.) for a personalized experience.
Incorporate other stakeholders' unique needs for Trace​
​
Provide views that are tailored to other stakeholders (e.g., PMs) and allow for further customizability on the platform