Needfinding
Machine
Makes contextually-situated data collection at home possible

No Tools Are Designed to Collect Real-Time Data Remotely
The UX community was caught off guard: UX researchers' data collection was often completely halted due to COVID.
In 2020, COVID-19 threw everyone off course. The abrupt change introduced by social distancing forced almost everyone to start working remotely overnight. The challenges the UX community faced pointed to a critical shortcoming of current tools: the inability to gather contextually-situated data remotely.
We wanted to design a system that empowers automotive UX researchers to leverage remote research to collect contextually-situated data in real-time.
On this project, I was one of the two researchers to identify pain points and translated user needs to design decisions. Then, I was the sole designer who designed and iterated on all the features, UI, and interactions of the design.
Role
UX Researcher & UX Designer
Duration
9 months (Jan-Sept 2020)
Responsibilities
Research, Interaction Design, UI Design
Team
Judy Chun, Nik Martelaro
How might we augment automotive UX practitioners’ capabilities by allowing them to collect contextually-situated, real-time data remotely?


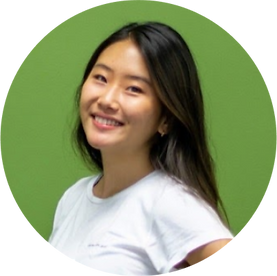
Duration
9 months
Roles
UX Researcher & UX Designer
Responsibilities
Research
Interaction Design
UI Design
Team
Chi Huang
Judy Chun
Nik Martelaro
Challenge
In 2020, COVID-19 threw everyone off course. The abrupt change introduced by social distancing forced almost everyone to start working remotely overnight. Yet, the sudden, drastic shift in work styles led to a lot of challenges.
We wanted to examine how the UX community is coping with the situation, the challenges that they are facing, and how we can build a system that really leverages remote research to address unmet needs.

Solution
Needfinding Machine is a remote data collection tool that empowers automotive UX practitioners to seamlessly collect less biased, contextually-situated data in real-time.
Contextually-Situated Data Collection
1. Easy to Understand Visualizations
Needfinding Machine shows-in-the moment the following:
-
The car's speed
-
The car's rotation
-
When the driver hits on break
-
The car's location on the map
-
Metadata of the location (e.g., pedestrian-heavy zone, traffic-heavy, zone, and etc.)
It accounts for the needs of both quantitative and qualitative UX professionals by visualizing complex information in easily digestible ways.
The visualizations better allow users to put themselves in the participants' shoes and ask appropriate contextually-situated questions remotely.
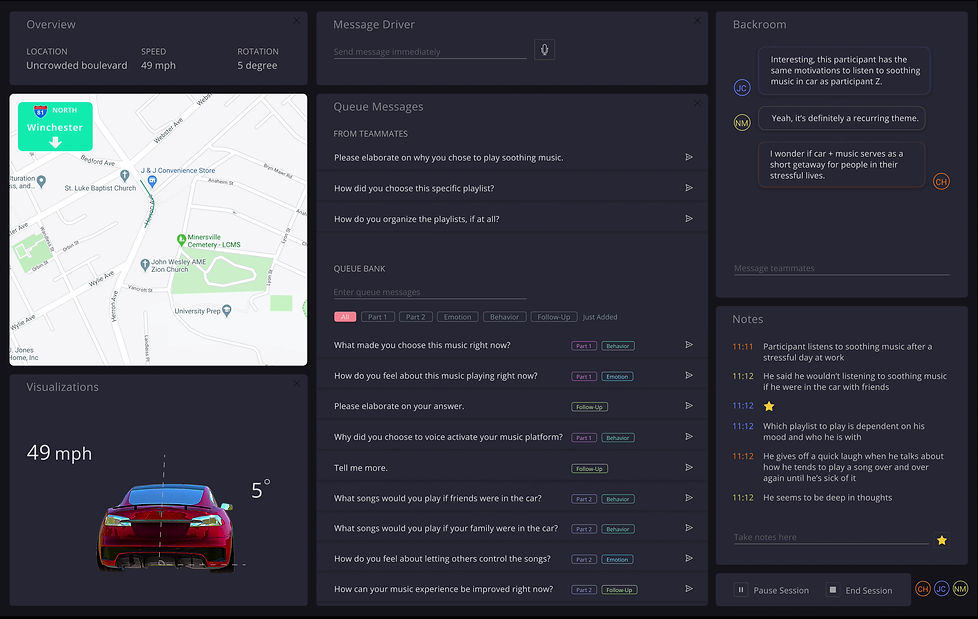
2. Communicate via a Bot Voice
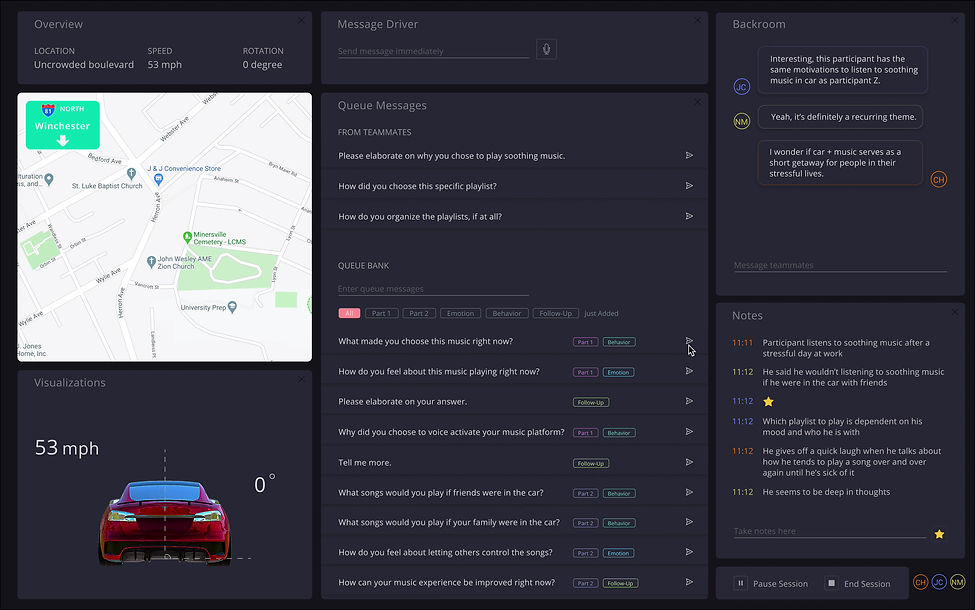
Users are able to communicate in a bot voice via the following:
-
Queue messages
-
Speech-to-Text
-
Type and send messages in real-time
Contextually-situated research is usually UX professionals' most loved method because it yields the most valid, close-to-real-life results. Yet, they often voice the concern of the participant changing his/her behaviors in the presence of another person.
Needfinding Machines decreases the possibility of social desirability bias by allowing UX professionals to interact with the participant via a bot voice.
Transparent, Collaborative, and Efficient
To ensure seamless collaboration and efficiency, Needfinding Machine supports the following:
-
A backroom channel that allows the main facilitator to communicate with other researchers/observers
-
Other researchers/observers are able to queue messages as appropriate
-
Real-time color-coded notes that show are the notetakers
-
Notes are time synched to the recordings
Research is very much a collaborative effort and the best ideas come about when multiple people communicate and contribute.
Needfinding Machine makes collaboration extremely easy and organized by allowing other researchers to queue messages to the participant, and the main facilitator acting as the moderator and determining which messages actually get sent.
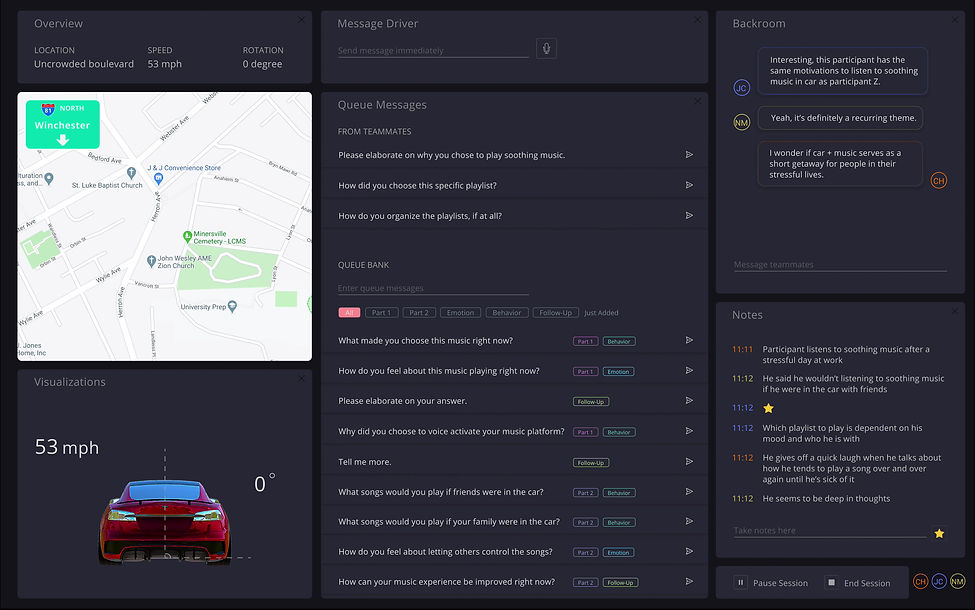
Customizability
1. Tailored Notifications
Users can customize the following notifications to ensure more efficient, targeted data collection:
-
When the car speed increases/decreases by a certain mph
-
When the car speed changes by a certain mph in X timeframe
-
When the car rotation increases/decreases by a certain degree
-
When the car rotation changes by a certain degree in X timeframe
-
When the car enters an area of interest
One interesting aspect about contextual research such as drive-alongs is that the majority of the time the session is uninteresting to the UX professionals. They're waiting for the moments, which are often far and between, that are interesting to their research goals.
Needfinding Machine allows automotive UX professionals to conduct more efficient research by notifying them when a phenomenon of interest occurs in a highly dynamic and constantly changing context.
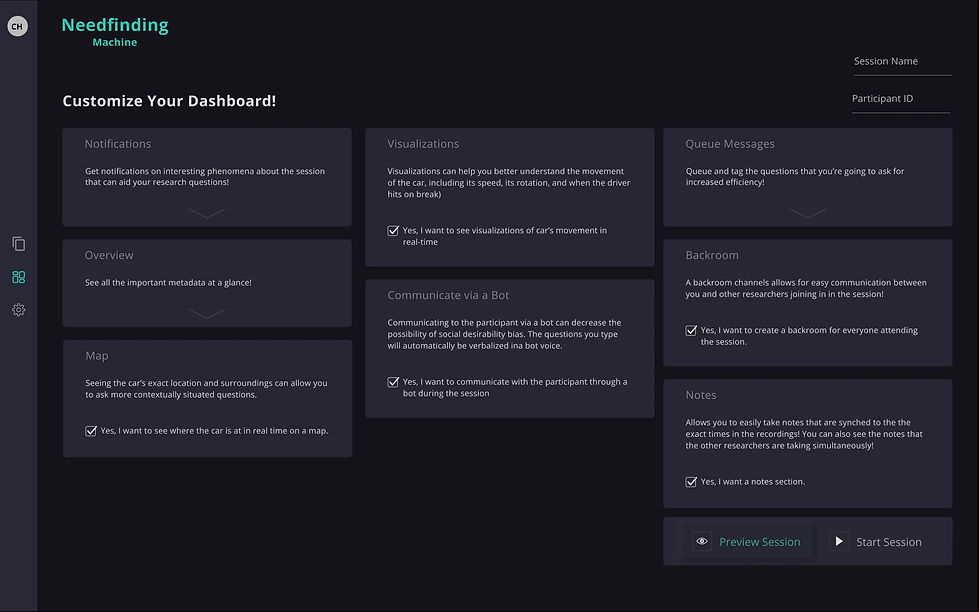
2. Queue Questions for Increased Efficiency
Needfinding Machine allows users can do the following with the queue function:
-
Queue and tag questions
-
Quickly filter via tags
-
Reuse previous questions
One utmost importance of remote research is immediacy—how fast can the UX professional asks the question of interest at the appropriate moment.
Our design not only allows users to queue questions before the session begins, but also they can quickly filter the queue questions by tags during the session to ask the questions as promptly as possible.

3. Customize Dashboard Based on Needs and Goals
Based on users' preferences and research goals, they can customize the following:
To ensure maximum flexibility for automotive UX professionals, Needfinding Machine has several customizable functions to fit their unique needs and projects.
-
What metadata gets displayed in the "Overview" Section
-
What to receive notifications of (speed, rotation, geofence)
-
What sections show up on their dashboard
-
The size of each section
-
The location of each section

Exploratory Research
Survey
Overview
When COVID-19 hit and everything became remote, we wanted to study how UX research professionals are responding to recent moves to remote work during the outbreak.
By understanding their tool usage for remote work and the strengths and shortcomings of those tools, we can better design a tool that addresses the gap between their needs and the tools available. Specifically, we're looking at three main phases of their workflows: planning research, conducting research, and analyzing research.
Goals
-
Understand how COVID-19 has been impacting researchers' workflow
-
Pinpoint how they are adapting and what (combination of) tools they are using to adapt to remote research
-
Dive deeper into the challenges and workarounds that they are employing
-
Explore the similarities and differences in terms of challenges faced across different regions and industry sectors
-
Examine what they wish to get out of with remote research that they currently are not able to
Survey Results
-
Sample Size: 56
-
Target Group: User research professionals
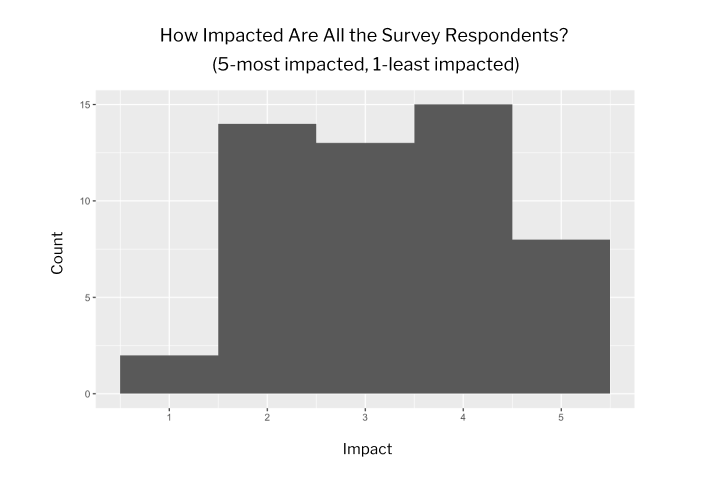
It's pretty evenly distributed in terms of how impacted the UX professionals are by the pandemic.
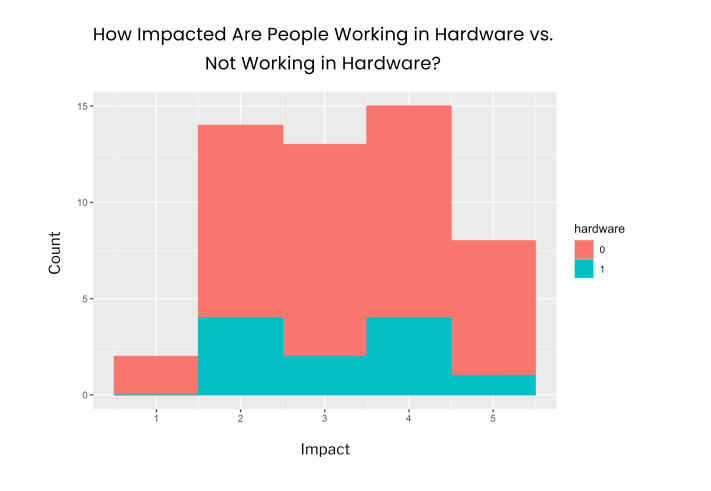
This plot suggests that there really isn't much of a difference between hardware and not hardware in terms of impact. But all the people who worked in hardware also worked in software in our sample, which may explain why there aren't major differences.
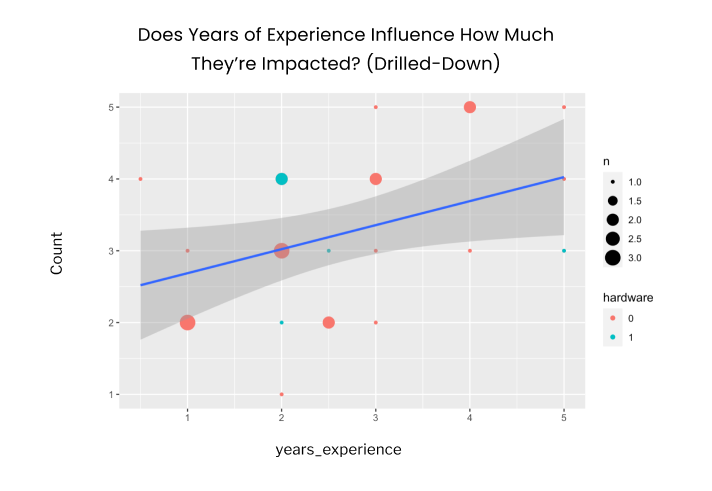
There's statistically significant correlation between years of experience and how impacted they were, given that the researchers had less than 5 years of experience. When they have experiences closer to 5 years, they're impacted more compared to their less experienced counterparts.
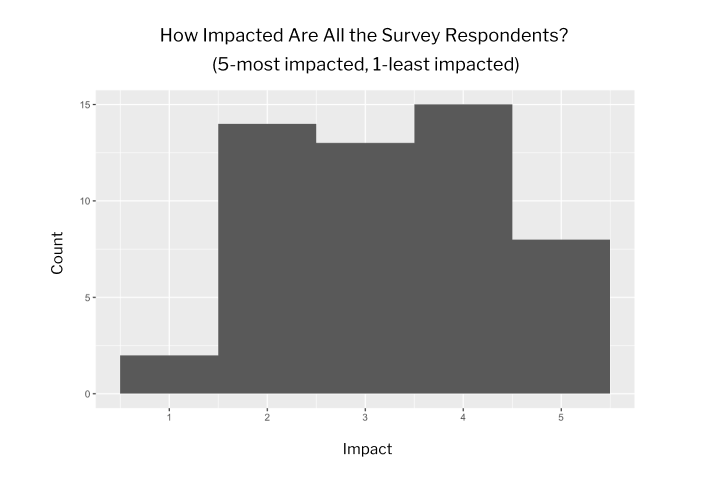
It's pretty evenly distributed in terms of how impacted the UX professionals are by the pandemic.
Insights
1. Most data collection tools don’t give them contextually-situated answers, which calls into question the validity of the data gathered.
“There's a lot of communication and understanding that happens in a physical space beyond just voice and looking at someone's face."
2. The lack of spontaneity and synchronous communication hinder collaboration and the sparks of creative ideas.
Work from home hinders the opportunity for spontaneous conversation and instantaneous question-and-answer, which are often sited as the source for great ideas.
4. The lack of integration between tools and/or incomplete functionalities offered by the tools lead researchers juggling between multiple tools to fulfill their goals.
Research professionals rely almost solely on digital tools now. But since there’s no single tool that suits their entire workflow and the softwares that they use are oftentimes incompatible, it has led to a lot of annoyances, confusion, and inefficiency.
3. In the three main phases of their workflows—planning, collecting, and analyzing, planning research was least impacted by the pandemic.
The UX community heavily relied on in-person methods for data collection and analysis prior to the pandemic. Hence, there's a rougher transition to adapting new tools and workarounds for those workflows.
Interviews
Overview
In addition, automotive UX researchers are among those most impacted by COVID-19. Since they often rely on gathering contextually-situated data in cars to fuel their design directions, the research methods that they utilize are heavily dependent on being in-person.
We wanted to study the extreme of the spectrum—the automotive UX researchers—to examine in-depth how they’re currently partaking the three major stages of research: planning, collecting, and analyzing data.
Goals
-
Understand the workarounds that researchers rely on when working around the challenges of remotely researching
-
Identify what researchers can get out of in-person research that they can’t achieve with remote research and vice versa
-
Pinpoint the new tools that the researchers are employing in their workflows to achieve their research goals

Details
-
Sample Size: 14 participants
-
Titles: UX Research Assistant, UX Researcher, Senior UX Designer Research Manager, Designer/Design Researcher, Research Scientist
Affinity Diagramming from the Interviews
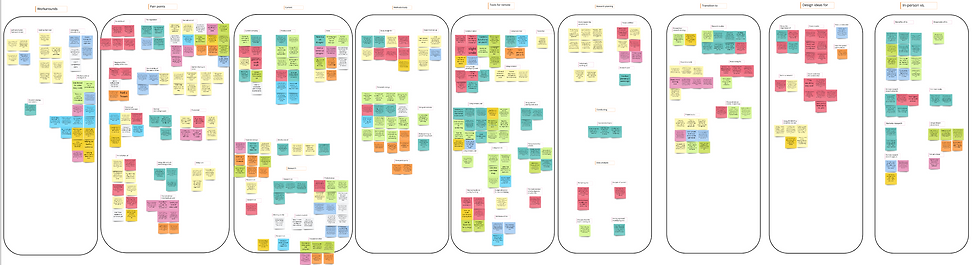

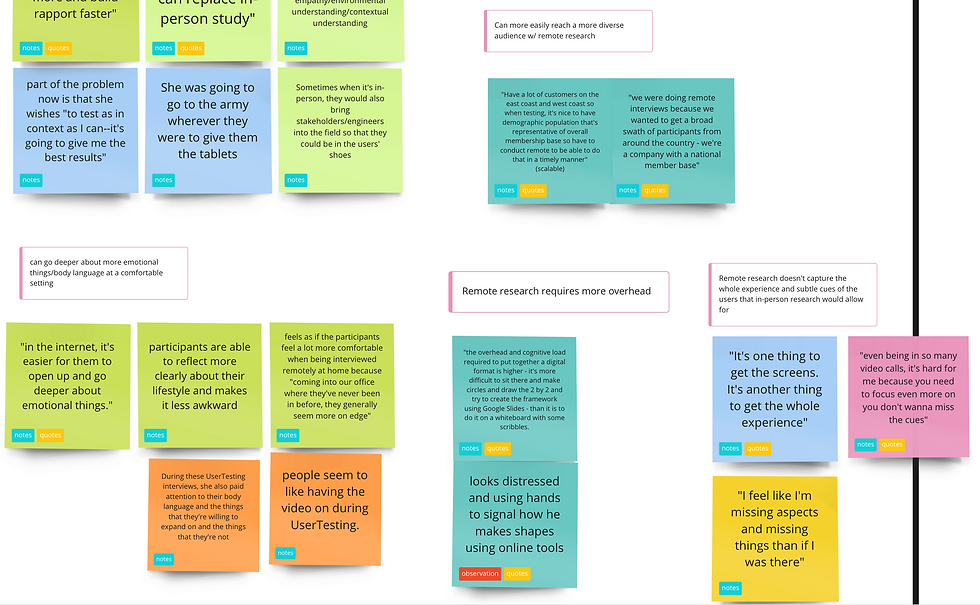
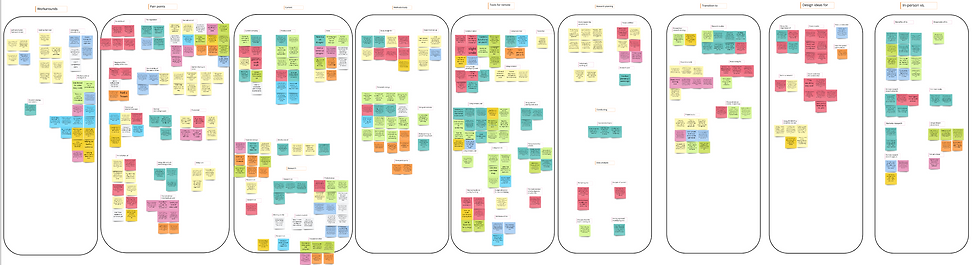
Insights
1. Immediacy, accuracy, and empathy-building are the main factors that make in-person research so appealing. Yet, they’re forced to shift to asynchronized and user-paced research.
Being able to observe and interact with participants with no delays, submerge themselves in participants’ environment, and hence, build empathy in the process are the three crucial benefits of drive-alongs and contextual inquiries.
2. Studies that require physical access to cars have been severely hindered, as no tools are available to capture the contextual environment and situational factors that are so critical for in-car experiences.
Automobile experience is especially influenced by situational factors. It’s hard to replicate how participants would feel or react in a real vehicle even with AR/VR or simulated environment.
3. Workarounds to having no in-person research is to use remote tools for experience sampling.
Diary studies and participants video recording snippets of their journeys are used as alternatives to in-person field work.
4. Focus is shifted away from execution and more towards research planning, research analysis, and tangential work.
Researchers allocate the time that has been freed up from data collection to more research planning (e.g., in-depth literature review) and analysis to ensure that the research design and insights are solid.
Diary Study + Follow-Up Interviews
Overview
In order to get more contextual, in-the-moment data regarding how the automotive UX professionals are employing different tools to achieve their tasks, we decided to conduct a one week long diary study.
Details
-
Sample Size: 5 participants
-
Length: 7 days
-
Technique: Reflection Period & Short Snippets
Participants are asked to reflect on their usage of tools at the end of each day (“Reflection Period”). In addition, if there’s a salient experience, they can also choose to report it in-the-moment (“Short Snippets”).
Goals
-
Understand their usage of tools regarding three instances of remote work: planning, conducting research , and analyzing data
-
Discover key micro-moments and interactions that pushes them to frustrating or delightful moments
-
Explore how the usage of tools or pattern of use differs when remotely working individually vs. collaboratively
-
Get a closer look at the nuanced thoughts and attitudes that impact the researchers’ tool usage decisions
-
Analyze the sentiment associated with each tool usage
-
Plot out new design features for tool based on underlying needs/challenges that they face and prioritize design opportunities grounded on user needs
Affinity Diagramming from the Diary Study
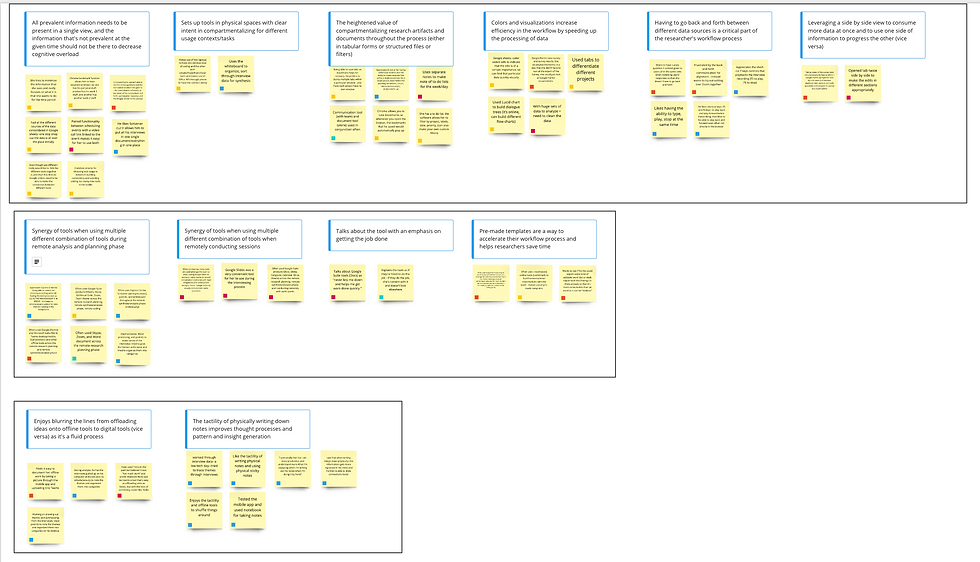
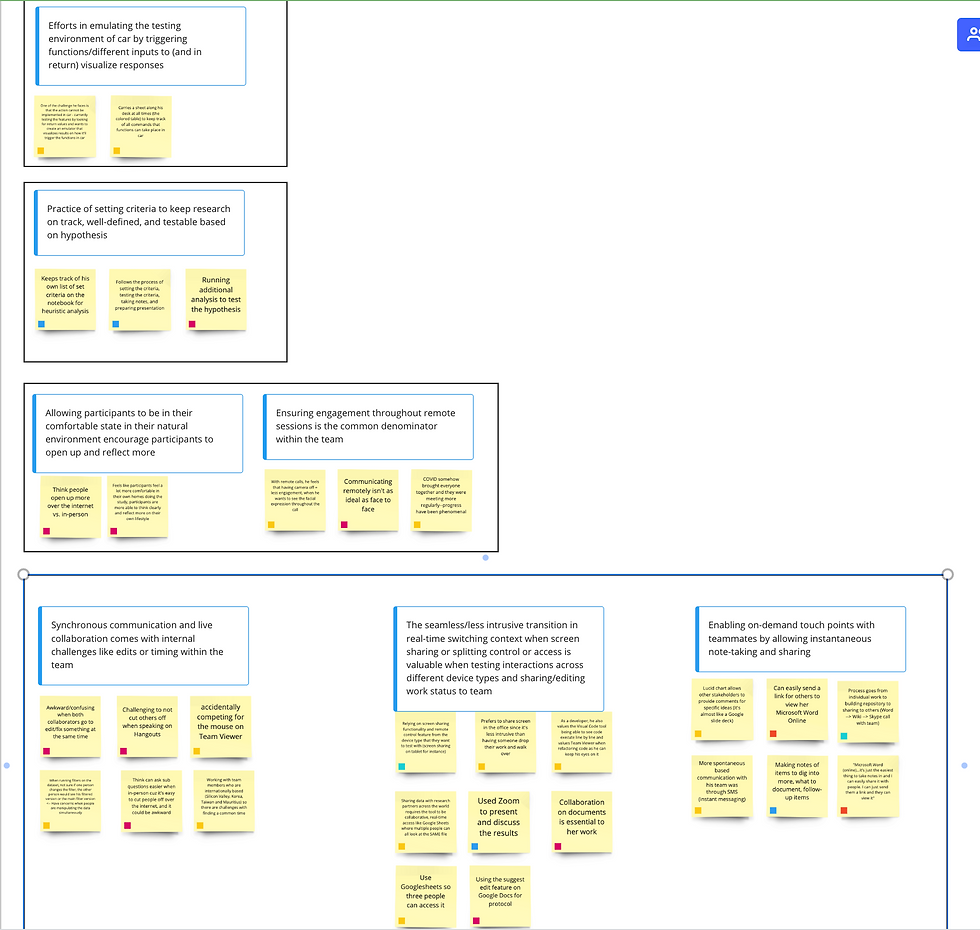
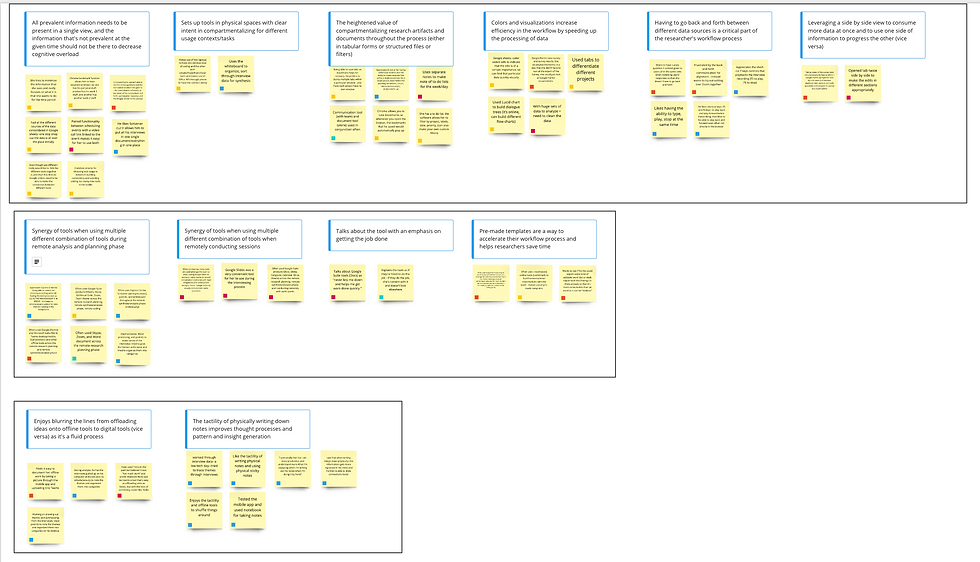
Insights
1. All prevalent information needs to be present in a single view, and the information that's not prevalent at the given time should not be there to decrease cognitive overload
Researchers compartmentalize different artifacts and incorporate colors, visualizations, and filters to increase work efficiency and reduce cognitive load.
2. Researchers often use synergy of tools synchronously to customize to their needs to complete each phase--planning, conducting, and analyzing.
E.g., For conducting research, one researcher reported using Google Docs for protocol, voice memo to record conversation, Zoom to talk to the participant, and Google Slides to visually demonstrate some questions
3. Synchronous communication and live collaboration comes with internal challenges like edits or timing within the team.
Synchronous communication is crucial, yet it increases the possibility of awkward and confusing interactions. E.g., collaborators fixing/overriding something at the same time, cutting others off on video call, and competing for the mouse on Team Viewer.
4. Seamless collaboration and familiarity to the tools by different stakeholders often drive which tools are chosen to complete the tasks.
Researchers often default to using tools that require minimal onboarding costs like Google Suite tools when the endeavors require the involvement of different stakeholders.
5. There’s heightened value to tools that enable on-demand touch points with teammates by allowing instantaneous note-taking and sharing.
Their work is often collaborative, and they work in a larger ecosystem of teams and stakeholders. Thus, it’s essential that the platforms that they use to document ideas can be easily shared and commented on.
Concept Generation
Storyboards
Overview
We’ve learned that the biggest challenge for the UX community amidst this pandemic is the ability to conduct research that’s situated in the context.
For the automotive industry especially—a field that heavily relies on in-person research, such as drive-along and contextual inquiries—has been severely impacted.
We wish to target their biggest pain point by designing a solution that allows automotive UX professionals to conduct contextually-situated research remotely. The solution will take into account the common pain points and work styles that emerged from the synthesis of previous research methods.

Goals
We now want to (in)validate automotive UX professionals' underlying needs when they conduct research by storyboarding.
Specifically, we looked at four main topics:
-
Moderated remote data collection
-
Unmoderated remote data collection
-
Mixture of moderated and unmoderated
-
Data sense-making/Interface design
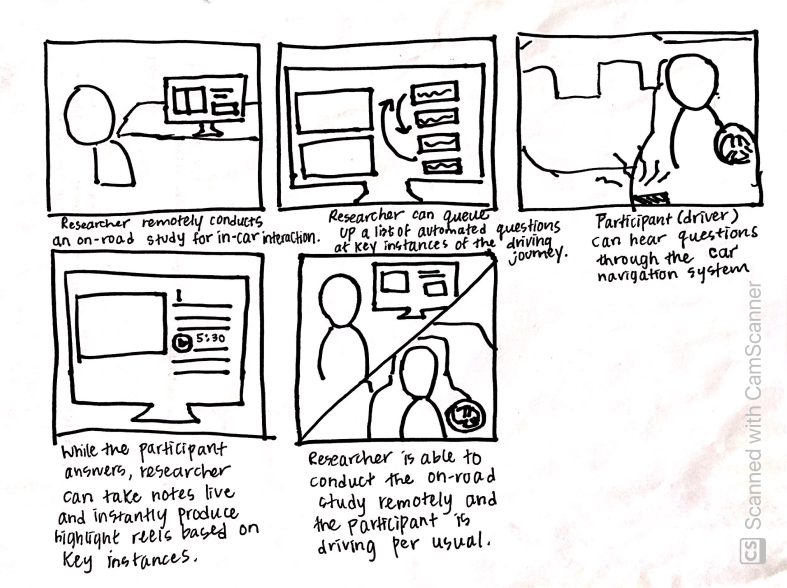
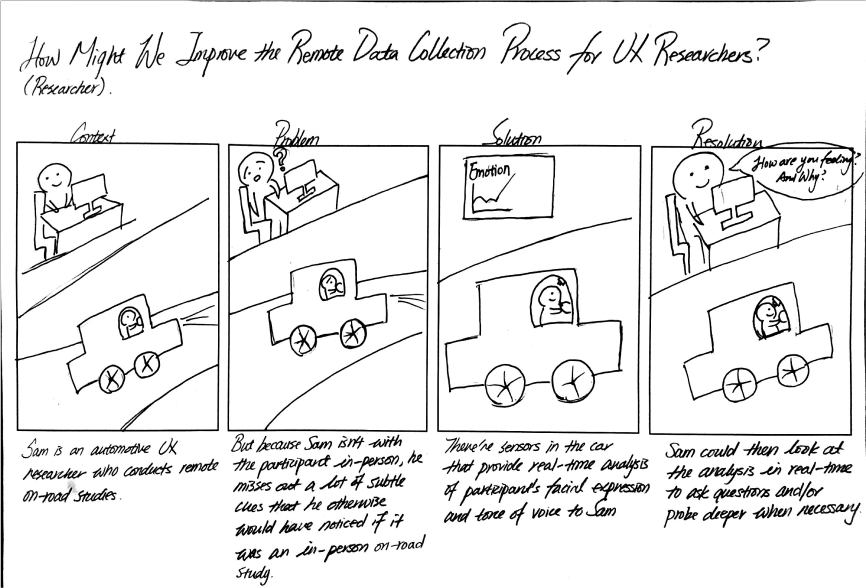
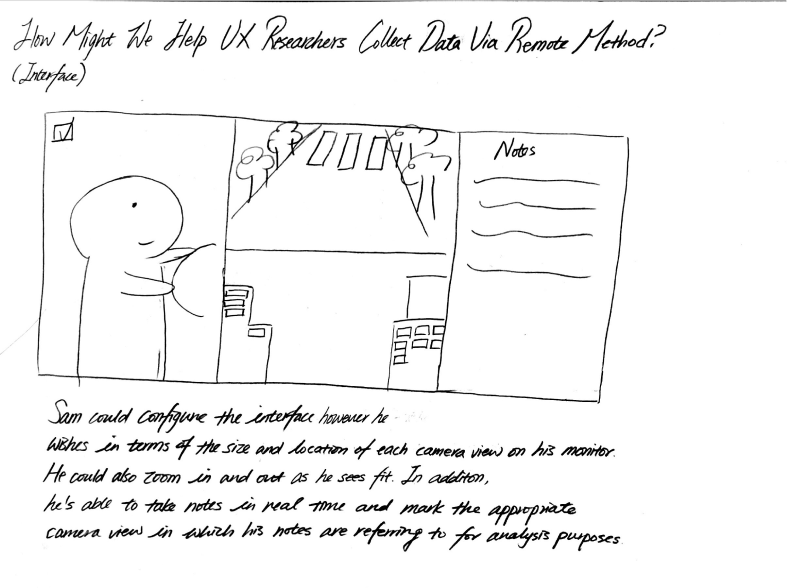
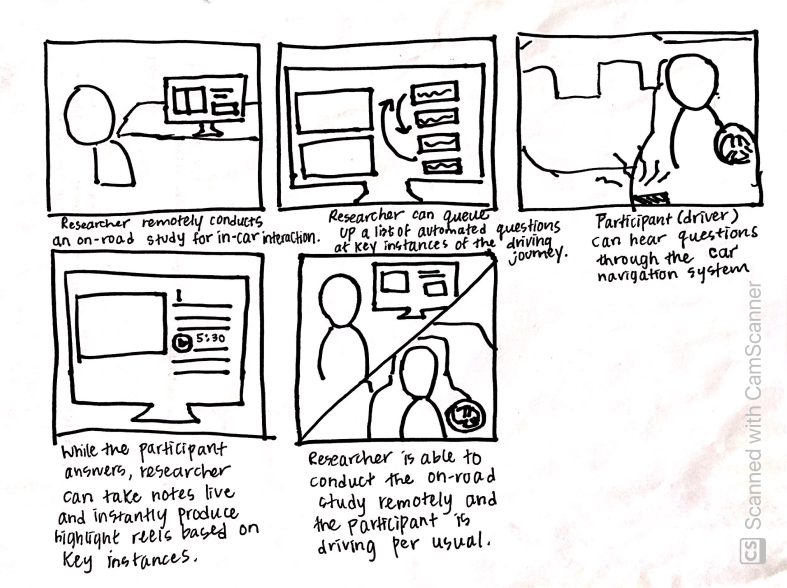
Insights
1. Researchers are concerned that asynchronous research might not capture contextual cues, yet there’s an inherent distrust in the tools to detect subtle cues for synchronous research.
Researchers voiced that they trust themselves or their teammates more than a machine in detecting subtle cues (e.g., facial expression analysis).
2. Some information and data visualizations may be useful but is difficult to comprehend and overwhelming for researchers who only do qualitative research.
Designing an interface where qualitative researchers can also understand and incorporate quantitative data streams to strengthen their research and findings will be immensely useful.
3. Even though researchers have a strong need for contextually-situated data, they are concerned that the presence of a researcher will bias the actions of the participant.
One of the biggest cons of an in-person research is that participants might unconsciously alter their response or actions, which put the validity of the data gathered into question.
4. There’s a need to confirm their observed phenomenon with different sources of data. More triangulation of different data streams lead to a firmer belief that their observation is correct.
E.g., when a researcher wants to study attentiveness, he/she wants to examine various factors, such as posture, car’s surrounding, action, and eye gaze that all might indicate/influence attentiveness.
5. Having the ability to ask follow-up questions to probe deeper are essential, as most insights arise from going deeper than the standardized questions.
Though having automated questions can decrease the researchers’ workload and scale up their research efforts, having certain level of customizability and ability to intercept are crucial.
Evaluative Research
Simulated Remote Drive-Along + Follow-Up Interview + Storyboarding
Overview
From the previous storyboarding sessions, we’ve pinpointed user needs and the features that could assist them.
But in order to ensure that the interface that we’re designing addresses their needs for a contextually-situated, remote data collection tool, I wanted to create a “simulated remote drive-along.”
Goals
The study will allow us to see in real-time how the researchers use and interact with the tools that we provide to conduct a remote drive-along and the tools’ strengths and shortcomings.
Then immediately after the remote drive-along session when the researchers just experienced what it’s like to conduct remote drive-alongs, I wanted to do another storyboarding session. But this time, it is more feature-based than needs-based—to (in)validate the features of our design’s interface that best serve their needs.
Study Composition
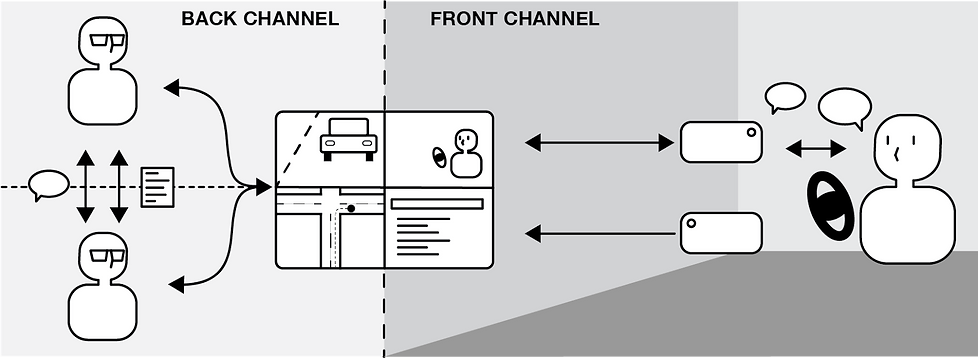
-
Researcher conducts the remote session
-
Zoom is used as the front room channel where the researcher can see two camera
-
Researcher communicates with the participant via a bot
-
My teammates and I are the observers
-
Skype is used as the backroom where we communicate with the researcher
-
Observers use Grain to communicate with each other and take notes
Researcher's Computer 1 View

Researchers can use the above platform, built by Nik Martelaro, to message/queue questions, see the location of the car in real-time, and take notes.
In addition, Skype is used as the backroom for observers to ask the researcher questions.
Researcher's Computer 2 View
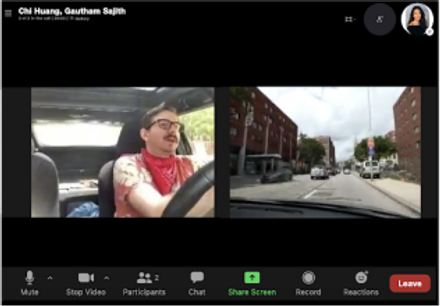
Zoom is used for the researcher to observe and ask the participant questions. The researcher can specify two camera angle views based on their research questions.
We use Zoom because when the call drops, I can text the participant the Zoom link, and the call can be automatically picked up.
Example of when the researcher uses the platform to communicate with the participant in a bot voice
Insights
1. To the researchers, the concept of “being contextual” means that the incoming data includes everything that the participant is seeing and experiencing in the moment, or else the data is not contextual—it’s arbitrary/inferior.
“I want to see everything and anything that the driver is seeing in order to ask the most appropriate questions”
2. In general, there’s no need to scale up research with completely automated questions, since they usually get the insights that they need from 6-8 participants.
The main purpose of scaling up research is to gain credibility with stakeholders because they tend to emphasize more on numbers. But researchers believe that for their own purposes, 6-8 participants are sufficient.
3. Simultaneously typing the questions/notes and listening to what the participant is saying require a lot of cognitive attention, which might lead to missed information.
Researchers reported that they feel like they were missing a lot of potential important information because their attention were pulled by two very different sensory modalities with typing and listening.
4. More contextual cues and high-level information are needed when the researchers are unfamiliar with the areas in which the session is being conducted.
E.g., the map is not useful to the researchers when they're not from the area in which the participant is driving in since it only consists of street names.
5. Reducing the latency as much as possible is critical to remote synchronous data collection.
Every milliseconds count. Being able to ask questions immediately after an observed phenomenon occurred with little delays is absolutely essential.
Next Steps
Future Vision
1. Expedite the question-asking and insights generation process
Visualizations that compare the the current participant's incoming, real-time data with the accumulated/averaged data from previous participants would allow users to probe in the right direction.
2. Streamline the process from data collection to analysis
The ultimate goal of data collection is to be able to generate insights. Thus, incorporating machine learning to assist users to find recurring patterns or interesting phenomena across all participants to accelerate the data analysis phase will be hugely beneficial.
3. Encompass more functionalities that support experimental research
The interface currently allows for more exploratory research. In the future, it will be helpful to account for experimental research (e.g., allowing in-car remote music control, and the user examines participants' response/reaction)